Yoonseok Jang, INEEJI Business General Manager, gave a presentation on ‘The need to develop an AI-based optimal energy prediction/control system’
“The energy cost burden on companies is increasing due to the high volatility in the industry... “Using AI is meaningful”
AI-based cement firing process optimization and electric furnace operation optimization case “Selected as a government task”
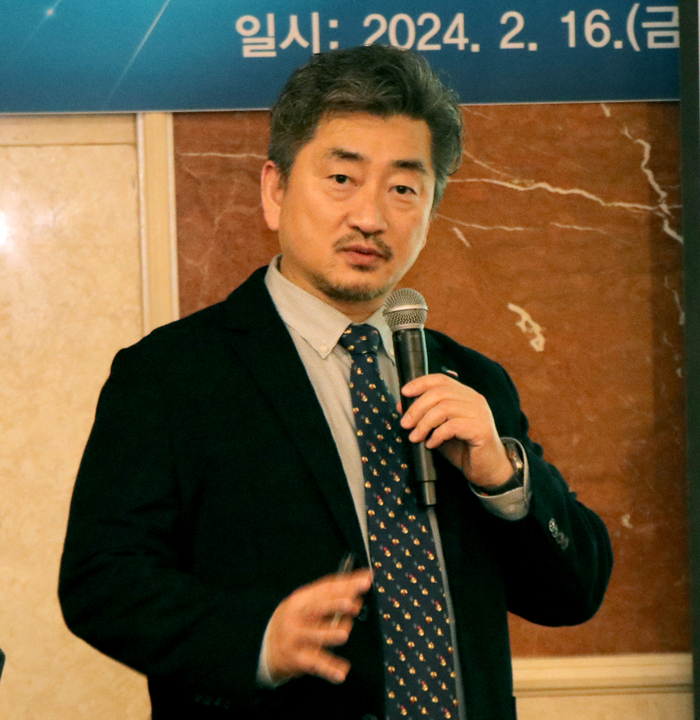
“AI technology should be introduced in areas that can be improved. Changing high-efficiency equipment is expensive, so from an efficient perspective, we need to reduce production input and obtain maximum output through AI.”
Yoonseok Jang, general director of INEEJI business, attended the seminar 'Use of AI intelligent systems for efficiency of energy industry facilities' co-hosted by Energy Economics Newspaper and Energy Economics Institute at the Kensington Hotel in Yeouido on the 16th, and spoke about 'AI-based optimal energy prediction and control.' Through a presentation on the topic ‘Necessity of system development’, the need to develop an AI-based optimal energy prediction and control system was revealed.
Director Jang said, “The industrial sector consumes 60.6% (two-thirds) of the country’s total energy consumption,” and argued, “It is necessary to secure energy reduction measures in the industrial sector from the economic and energy security perspectives.”
At the same time, “There are many changes taking place, such as a lot of GVC (Global Value Chain) reorganization and an increase in reshoring demands. “There are structural issues in the industry, so there is a lot of volatility in energy use,” he said. “Companies want to use as little energy as possible. There are ways to increase efficiency by replacing high-efficiency facilities and strengthening insulation, but using AI is meaningful.” He explained.
Director Jang said, “There are concerns that AI may take away jobs from an industrial perspective, but in reality, there is a shortage of people as the workforce structure has changed over the past 20 years. Energy costs have also risen so much in the past two years that the burden on companies is increasing.” “He said.
Director Jang emphasized that manufacturing costs should be minimized and added value should be maximized, and introduced six cases of AI-based optimal energy control.
The first is an example of building an AI-based #3CGL smart factory model line. Depending on the operating conditions, it was difficult to manage the current condition of cold rolled steel sheets after heat treatment, but by applying a process quality status prediction model and control model created with AI technology, quality was stabilized and fuel was successfully reduced by 3%.
The second is an example of optimizing temperature control in an AI-based glass melting furnace. When melting glass, the temperature rose to over 1000 degrees and the internal condition could not be directly determined, so fuel was used excessively. With the introduction of AI technology, the temperature of the glass melting furnace became predictable, reducing fuel usage by 3%.
The third is an example of optimizing the AI-based cement firing process. Energy costs and carbon dioxide emissions were high due to the use of bituminous coal when processing limestone. Recycled fuel (waste plastic) was used as an alternative heat source, but control was difficult due to quality imbalance. AI technology made it possible to predict changes in the state of the process and control them in advance, resulting in a 5% reduction in bituminous coal usage.
Director Jang said, “As we are seeing good results, the Ministry of Trade, Industry and Energy started it as a task and is spreading it.”
The fourth case was Bucheon City. Bucheon City experienced traffic congestion, but with AI technology, daily traffic increased by 5% without expanding the manufacturing process or infrastructure. As optimal signal control by AI becomes possible, it has the effect of reducing vehicle fuel use during congestion and reducing costs and carbon emissions.
The fifth case is optimizing diesel production in the RHDS process. It took time to confirm the quality analysis results at the factory, and real-time control until confirmation of the results was difficult, but with AI technology, the company succeeded in maintaining target quality, improving productivity, and reducing costs such as energy by 1%.
The last example is an example of optimizing electric furnace operation by predicting melting time using scrap metal composition and weight through AI technology.
When recycling metal products, scrap iron was melted in an electric furnace to make molten iron, but control was difficult because accurate state measurement was not possible due to changes in the scrap metal composition and the high temperature and pressure characteristics of the electric furnace. By introducing AI technology, we succeeded in reducing power use by 7.1%.
Director Jang added, “The results were so good that it was selected as a government project last year,” adding, “We are developing the technology by introducing it to a large steel company in Gunsan.”
He emphasized, “AI technology can be useful in many aspects,” and “It is a core technology that can be introduced into the process without additional facility investment, and it needs to be fostered through continuous R&D investment.”